Key
speakers
From Academic and Industry
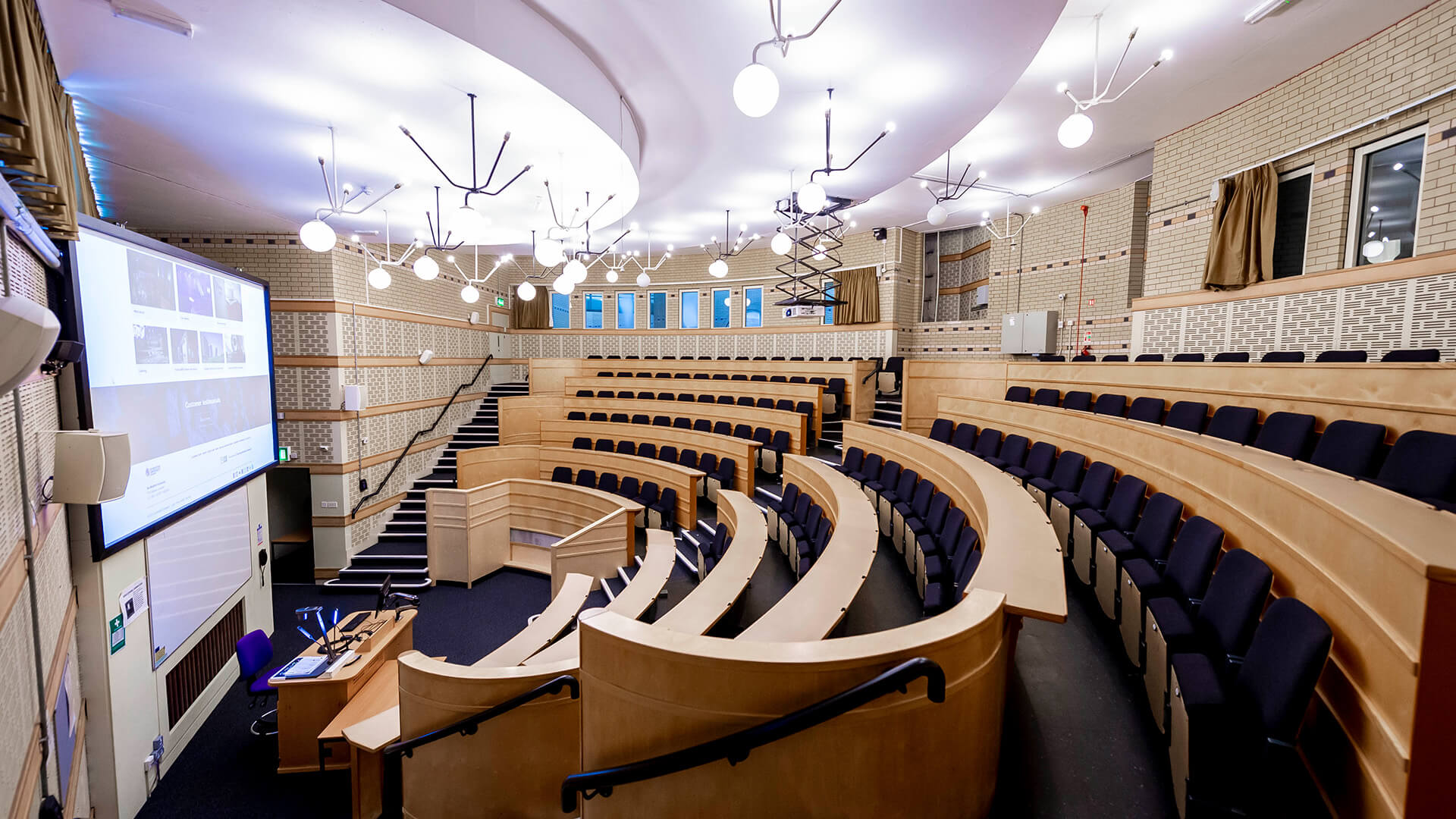
Discovering the nature through innovation and research
University of Aberdeen, UK
Title: Deep Learning and Simulations for Nuclear Reactor Anomaly Detection and Localisation
Abstract: The use of non-intrusive techniques for monitoring nuclear reactors is becoming more vital as western fleets age. As a consequence, the necessity to detect more frequently occurring operational anomalies is of upmost interest. In this talk, we will show how nuclear reactor core noise data can be employed to unfold from detector readings the nature and location of driving perturbations. Given that in-core instrumentation of certain western-type reactors are scarce in number of detectors, rendering formal inversion of the reactor transfer function impossible, this talk will describe the use of Deep Learning for the task of unfolding. We will discuss an approach to such a task doing so in the presence of multiple and simultaneously occurring perturbations or anomalies. A voxel-wise semantic segmentation network will be presented that can be used to determine the nature and source location of multiple and simultaneously occurring perturbations in the frequency domain. A nuclear reactor core simulation tool has been employed to provide simulated training data for two reactors. Lastly, we will talk about some pilot work towards the application of the aforementioned approach to real measurements, introducing a self-supervised domain adaptation procedure to align the representation distributions of simulated and real plant measurements.
Università Svizzera italiana (USI), Lugano, Switzerland.
Title: To be confirmed
Abstract: To be confirmed
Insitro
Title: Practical tools for privacy preserving synthetic data generation
Abstract: Synthetic data generation is quickly becoming a promising way to share sensitive data without violating data privacy. However, differential privacy (DP) based generative modeling strategies often lead to very poor sample quality, which constrains their downstream use. In the first part of my talk I will present privGAN, a novel generative machine learning framework which enables the generation of privacy-preserving synthetic data without sacrificing much downstream utility. PrivGAN focuses on protecting against a narrower notion of privacy than DP, namely the membership privacy risk, which is motivated by real-world privacy concerns in healthcare data sharing. This in turn leads to the generation of synthetic data which has a much better privacy/utility trade-off than DP based approaches.
In the second part of my talk I will present a novel membership privacy auditing framework for generative models, called MACE. While there has been much work on non-DP generative modeling since privGAN, there currently exists no formal way to quantify the privacy loss of such models. MACE poses membership inference as a classification problem and shows how Bayes optimal classifiers can be used to estimate the expected membership privacy loss of models for a given dataset. In addition to estimating membership privacy loss of models, our framework is also able to identify the membership privacy risk to individual samples.
Through the participation in research we are making a difference in the sustainable society.
CEM PGR conference organizing team
click on the button below to join the conference, to become a presenter please go to the Resigtrstion/Submission page.